[ad_1]
Giant language fashions (LLMs), like ChatGPT and Microsoft Copilot, have moved rapidly out of the novelty part into widespread use throughout industries.
Amongst different examples, these new applied sciences are getting used to generate buyer emails, summarize assembly notes, complement affected person care and supply authorized evaluation.
As LLMs proliferate throughout organizations, it turns into essential to judge the place we are able to apply conventional enterprise processes to watch and make sure the accuracy of the fashions.
Let’s take a look at the function that enormous language mannequin operations (LLMOps) can play within the explosion of LLMs in enterprise and focus on further challenges that LLMOps may assist remedy.
A fast background of MLOps
Monitoring machine studying fashions after they’ve been put into manufacturing has lengthy been an essential a part of the machine studying course of. Whereas nonetheless quick evolving, the sphere of machine studying operations (MLOps) has come into its personal, with giant and lively on-line communities and plenty of knowledgeable practitioners.
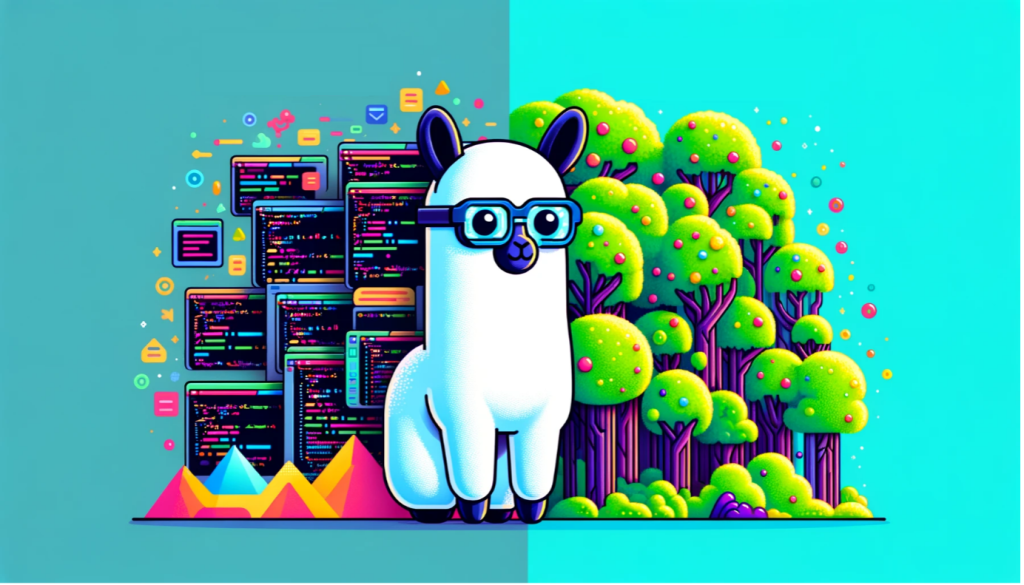
The worth and wish for MLOPs are clear since fashions in manufacturing are inclined to degrade over time as consumer habits shifts. As these shifts happen, it’s essential for the enterprise to make sure consistency within the information and the standard of the fashions. The demand for documentation and verification of mannequin accuracy is on the rise resulting from regulatory initiatives just like the EU AI Act.
Like so many different areas throughout society, the introduction of ChatGPT in Nov 2022 modified the panorama of MLOPs. With increasingly companies utilizing LLMs, the subject of LLMOps is gaining traction. In spite of everything, if monitoring and sustaining fashions in manufacturing has worth for different varieties of machine studying, it absolutely has worth for LLMs, too.
Since then, LLMs have develop into indispensable instruments for professionals throughout varied sectors, providing unprecedented capabilities in pure language understanding and content material era. Utilizing these highly effective instruments in sensible functions introduces a specialised operational area: LLMOps. This idea extends past conventional machine studying operations, specializing in the distinctive challenges related to the deployment and upkeep of LLMs for end-users.
Navigating LLMOps: A consumer’s information
LLMOps embody the methods and practices required to successfully combine LLMs into enterprise processes. In contrast to conventional MLOps, which can cater to numerous machine studying fashions, LLMOps particularly addresses the intricacies of using LLMs as instruments somewhat than creating them.
The core challenges with LLMOps contain managing the unstructured nature of enter information and mitigating the dangers of deceptive or inaccurate outputs, generally known as hallucinations. Let’s have a look at every of these areas in additional element.
The problem of unstructured inputs
One of many major hurdles in using LLMs is their reliance on huge quantities of unstructured information. In sensible functions, customers typically feed LLMs with real-world information – together with photographs, textual content and movies – that may be messy, inconsistent and extremely different.
Knowledge can vary from buyer queries and suggestions to advanced technical paperwork. The aim for LLMOps is to implement methods that may preprocess and construction this information successfully, guaranteeing that the LLM can perceive and course of it precisely, thus making the software extra dependable and environment friendly for end-users.
Addressing output hallucinations
One other vital problem is the potential for LLMs to generate believable however incorrect or irrelevant responses, referred to as hallucinations. These inaccuracies could be problematic, particularly in important functions like medical diagnoses, authorized recommendation or monetary forecasting.
Right here, the aim for LLMOps must be to concentrate on methods to attenuate these dangers, akin to implementing strong validation layers, incorporating consumer suggestions loops and establishing clear pointers for human intervention when needed.
LLMOps vs. conventional MLOps
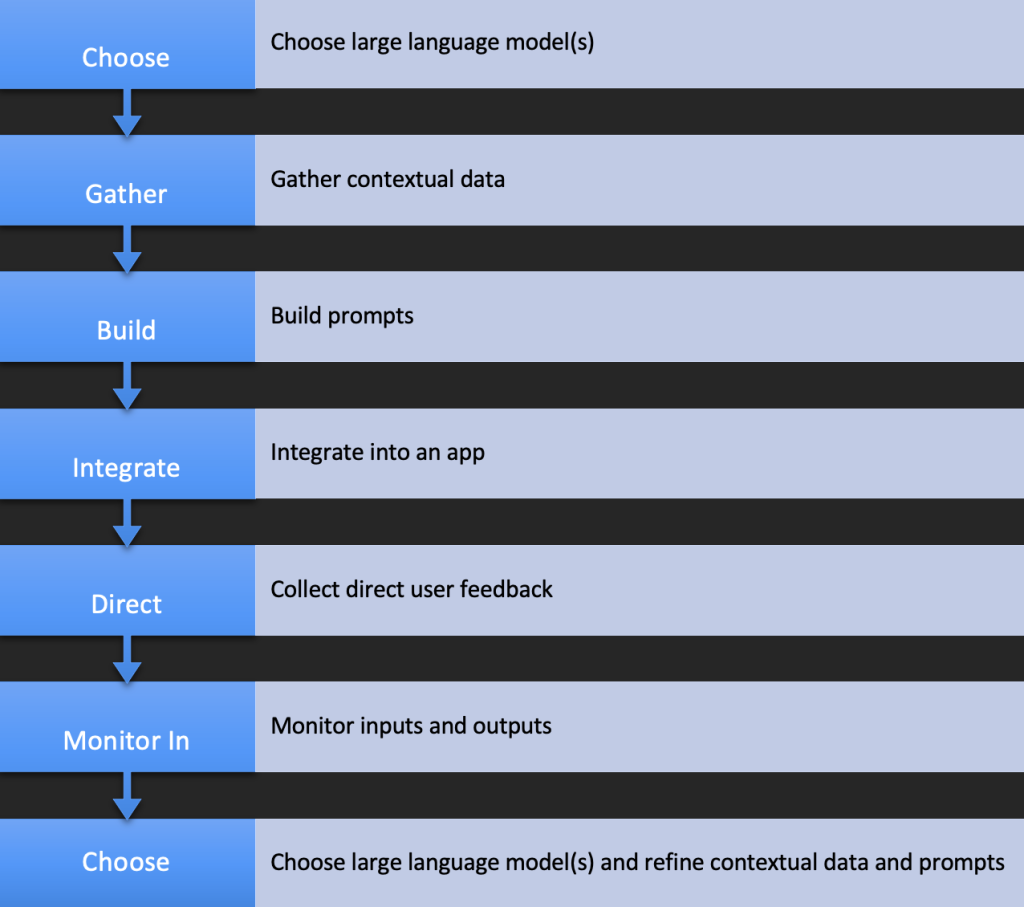
Whereas LLMOPs share some widespread floor with conventional MLOps – akin to the necessity for mannequin monitoring and efficiency monitoring – the main focus considerably shifts towards managing the consumer interplay with LLMs. The aim is to keep up the mannequin’s efficiency and make sure that the LLM is an efficient, dependable software for its meant software, accommodating the unstructured nature of human language and thought.
As LLMs proceed to permeate varied sectors, the significance of specialised operational practices can’t be overstated. LLMOps stands on the forefront of this new period, addressing the distinctive challenges of using LLMs as dynamic, interactive instruments. By specializing in the precise problems with unstructured information administration and output validation, companies can harness the total potential of LLMs, remodeling them from mere technological marvels into indispensable belongings of their operational arsenal.
Adapting to LLMOps just isn’t merely an operational improve however a strategic necessity for any group wanting to make use of the transformative energy of LLMs.
Learn: Generative AI and Giant Language Fashions Demystified
[ad_2]
Source link